700G
Background
Supervised machine learning models have been widely utilized for prediction and getting insight into diseases by classification of patients based on personal health records. However, a class imbalance is an obstacle that disrupts the training of the models.
Objective
We aimed to address class imbalance with a normalizing flow, one of the semi-supervised models for anomaly detection. It is the first introduction of the algorithm for tabular clinical data.
Methods
We collected data from 706 South Korean citizens, featured with genetic data obtained from direct-to-customer service (microarray chip), medical health check-ups, and lifestyle log data. Based on the health check-up data, six chronic diseases were labeled (obesity, diabetes, hypertriglyceridemia, dyslipidemia, liver dysfunction, and hypertension). After preprocessing, supervised classification models and semi-supervised anomaly detection models, including normalizing flow, were evaluated for the classification of diabetes, which had extreme target imbalance (about 3%), based on AUROC and AUPRC. In addition, we evaluated their performance under the assumption of insufficient collection for patients with other chronic diseases by undersampling disease-affected sample
Results
While LightGBM (the best-performing model among supervised classification models) showed AUPRC 0.155 and AUROC 0.832, normalizing flow achieved AUPRC 0.326 and AUROC 0.819 during ten evaluations of the classification of diabetes. Moreover, normalizing flow could outperform the supervised model under a few disease-affected data numbers for the other five chronic diseases.
Conclusions
Our research suggests utilization of normalizing flow when cases are insufficient for prediction of chronic diseases with personal health records.
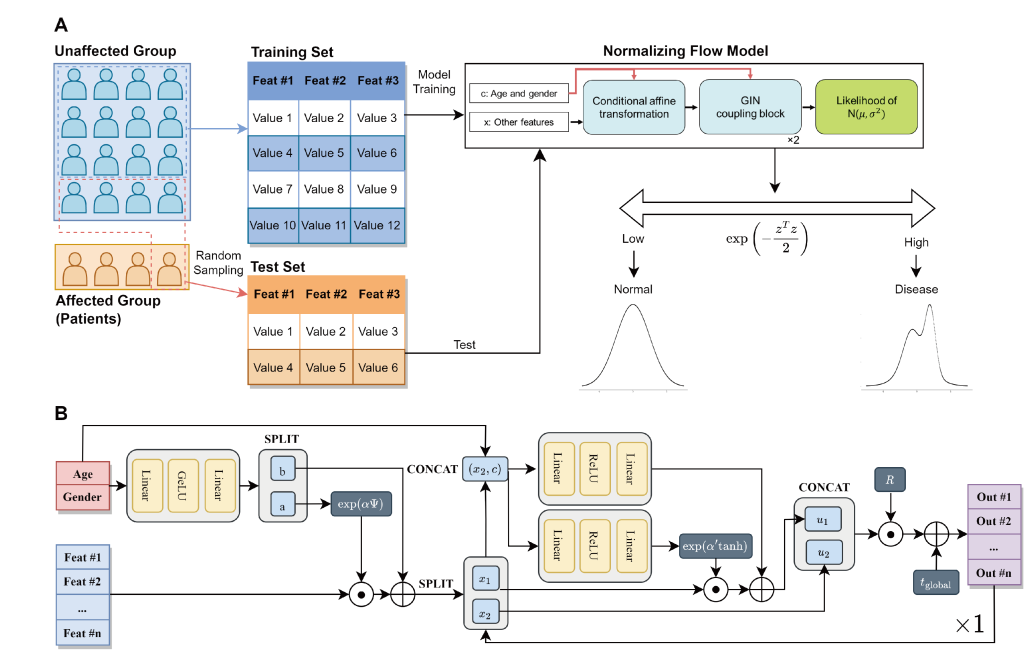