SplicingML
Genome-wide association studies (GWAS) have discovered enormous genetic variants associated with complex disease, including Alzheimer’s disease (AD), and these variants have contributed to a development of candidate preventive strategy and early detection biomarkers. Integrative analysis of multi-omics data plays a significant role in advancing our understanding in functional mechanisms of the AD-associated SNPs from GWAS. Through integrative analysis of genomic and transcriptomic data, disease-associated SNPs has been considerably reported as splicing quantitative trait loci (sQTLs) that affect alternative splicing exon alterations. However, most of GWAS studies have generated and analyzed genomic data, having not co-ascertained RNA-seq data, and it limits us to fully explore molecular mechanisms of GWAS-based disease-associated SNPs. In this study, we aimed to develop the predictive model that identifies genetic variants (sQTLs) affecting AS pattern changes from only genomic data by using machine learning techniques that have successfully allowed us to construct high accurate predictive models.
Why Alternative Splicing?
One enduring question is how a genotype contributes to a phenotype. We have seen dramatic advances in high-throughput technology, and high-throughput studies of biological systems are rapidly accumulating a wealth of ‘omics’-scale data. The development of Next Generation Sequencing technology is rapidly changing the face of the genome annotation and analysis field. We are now able to use genome sequence and mRNA expression data to improve our understanding of the pathogenic phenotype of human diseases or complex traits. There is a biological mechanism to relate the genome to the transcriptome. Short-term goal is to characterize this biological mechanism between these data that connect genotype to phenotype by focusing on alternative splicing (AS). Long-term goal is to create a molecular picture for genomics and personalized medicine. Our previous works have already developed the computational pipeline for integrating genomics with transcriptomics and providing functional annotation for intragenic SNPs involved in splicing regulation. The prominent works include incorporating these element into i) study of genetic basis of variations that affect splicing in human populations, ii) crosstalk between epigenetics and AS for exon recognition, and iii) resource generation for scientific communities. These resources harness the power of genome variation that facilitates enhanced understanding of its contribution to health disparities for diseases.
Multi-omics data integration tools
Genome-wide research has generated various data including multiple genome, transcriptome, epigenome, microRNAome, and proteome data, making it possible to conduct an integrative omics analysis. There exists clear recognition that the utilization of these multi-layered omics data is highly informative in understanding the complexity of RNA regulation. Therefore, we develops general resources that provide mechanistic information between DNA sequences and phenotypes through RNA regulation.
1. IMAS Integrative analysis of multi-omics data for alternative splicing (https://bioconductor.org/packages/release/bioc/html/IMAS.html)
2. CAS Visualization of Cancer Alternative Splicing (http://genomics.chpc.utah.edu/cas/)
3. ADAS Visualization of Alzheimer’s disease Alternative Splicing (http://genomics.chpc.utah.edu/AD/)
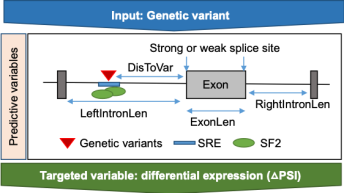